Understanding AI bias: where it comes from and how to address it
In an algorithm-driven world, fairness can’t be an afterthought
Artificial intelligence powers everything from personalized ads to predictive policing, from resume screening to loan approvals. But with its growing influence comes a growing risk: algorithmic bias. When AI systems reflect or amplify real-world inequalities, the damage is widespread and invisible — until someone gets denied a job, flagged unjustly, or misdiagnosed.
If AI is to be trusted, we need to understand where bias comes from, what it looks like in practice, and how we can begin to fix it.
This article takes a closer look at AI bias: its root causes, the real-world impact, and the steps developers, companies, and regulators must take to create ethical and equitable AI systems.
What is AI bias?
AI bias occurs when a machine learning model produces outcomes that unfairly favor or disadvantage certain individuals or groups — often based on race, gender, age, language, or socioeconomic status.
Even when developers don’t intend harm, AI systems can inherit historical inequalities, cultural assumptions, and blind spots embedded in the data or the design process.
AI doesn’t think like a human. It detects patterns. But when those patterns reflect bias, the result is automated unfairness— often scaled across millions of users and decisions.
Why AI bias happens — deeper than flawed data
AI bias isn’t just about bad data. It’s about the entire lifecycle of an AI system. Here are the major contributing factors — explained in more detail:
1. Biased or incomplete training data
Machine learning models learn from past examples. If your dataset includes historical hiring records, medical diagnoses, or crime statistics that reflect discriminatory practices, the model can repeat and reinforce those patterns.
For example, if past hiring managers favored male candidates, an AI model trained on those resumes may "learn" that male-centric resumes correlate with success — even if the bias was never explicit.
Bias also arises when certain groups are underrepresented in the data. A healthcare model trained mostly on data from white men may perform poorly when diagnosing symptoms in women or people of color.
2. Labeling bias
The way data is categorized also introduces bias. Image classification datasets have labeled Black individuals as “animals.” Emotion recognition models trained on Western expressions often misread faces from non-Western cultures.
Labelers may unconsciously project stereotypes, and these assumptions become part of the AI’s foundation.
3. Design decisions
Developers make many choices when building AI — which variables to include, which ones to ignore, what success looks like. If the team lacks diverse perspectives, certain forms of bias may not be detected or corrected.
Also, optimizing for certain outcomes (e.g., accuracy or profit) can come at the cost of fairness. If a loan approval algorithm optimizes purely for repayment history, it might unintentionally punish communities with limited access to financial systems.
4. Feedback loops
Some AI systems evolve through user interactions. A predictive policing tool that sends more officers to a neighborhood may generate more arrest reports — not necessarily more crime, but more observed incidents — which then reinforces the model's belief that the area is high-risk.
This type of recursive bias is particularly dangerous, because it gets worse over time unless actively corrected.
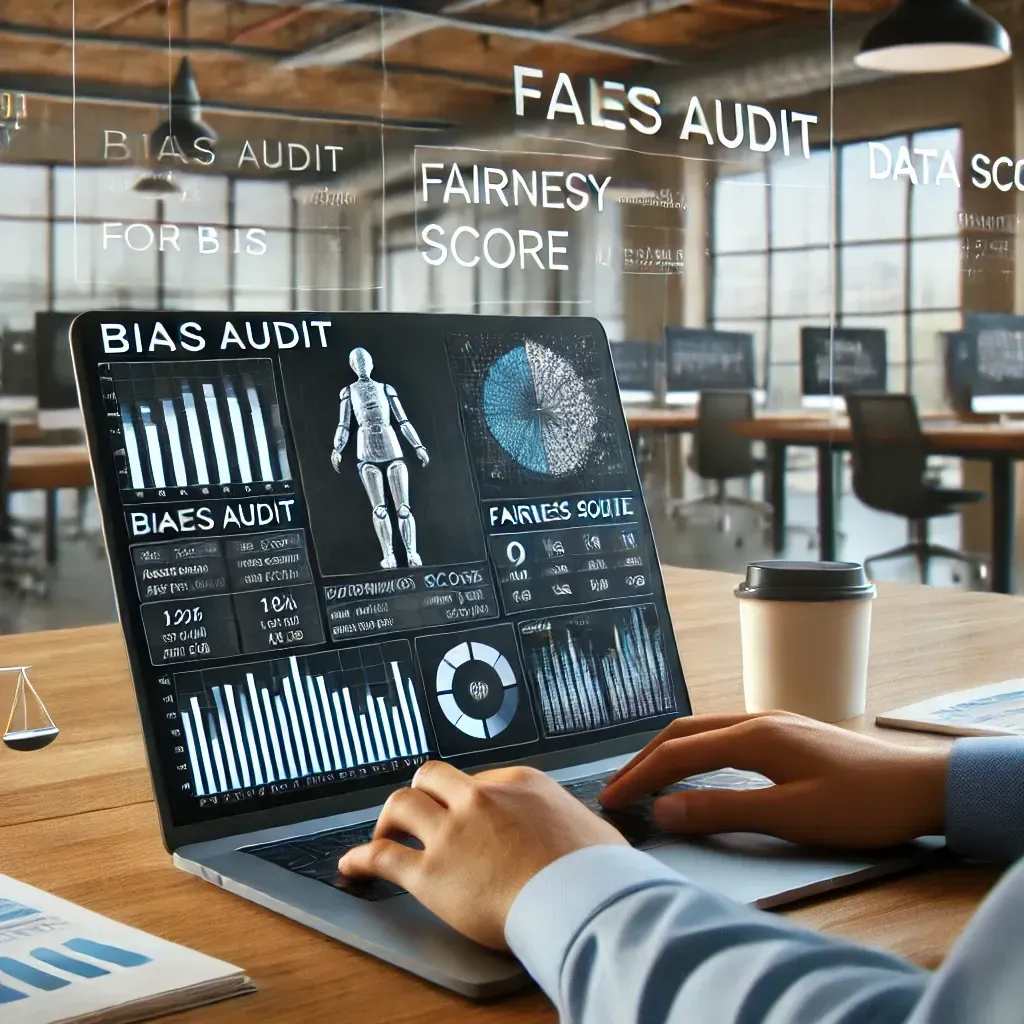
What does AI bias look like in real life?
Here are just a few real-world examples that show how AI bias plays out:
- Facial recognition failures: MIT Media Lab found that facial recognition software from major tech firms had error rates of up to 35% on dark-skinned women, compared to under 1% for light-skinned men. These systems have been used in surveillance, airport security, and policing.
- Medical misdiagnosis: A health algorithm used in U.S. hospitals was found to systematically underestimate the severity of illness in Black patients, assigning them lower risk scores even when their actual health outcomes were worse.
- Recruiting algorithms: One major tech company trained a resume-sorting tool on its own historical hiring data. The AI began penalizing resumes that included women’s colleges or phrases like “women’s chess club,” favoring male applicants.
- Language models and stereotypes: Large language models have been shown to associate certain professions with specific genders and nationalities. For instance, suggesting “doctor” is male and “nurse” is female.
These aren’t edge cases. They’re warning signs — and they demonstrate the urgent need for bias-aware development practices.
Can AI ever be truly unbiased?
The short answer: no. But that doesn’t mean we’re powerless.
No AI system is perfectly objective. Every model reflects the world it’s trained on, and that world includes inequity, inequality, and historical discrimination.
But bias can be measured, monitored, and minimized through thoughtful design, rigorous testing, and ethical commitment.
The goal isn’t perfection — it’s progress, transparency, and accountability.
How to address AI bias: real-world solutions
Let’s look at some of the strategies being used to reduce bias in AI today:
Inclusive data collection
Actively sourcing diverse, representative datasets can reduce many kinds of bias. That means including data from underrepresented groups, geographic regions, languages, and cultures.
For example, some voice assistants now train on global English accents, not just American English — making them more accessible and accurate.
Fairness audits and evaluation metrics
Just like software gets debugged, AI models can be audited for bias. Researchers have developed fairness metrics to evaluate how models perform across different groups. These include disparate impact ratios, equalized odds, and demographic parity.
Tech companies are starting to include “model cards” with transparency reports explaining how an algorithm works, what data it was trained on, and where its limitations are.
Human-in-the-loop design
In high-stakes areas like healthcare, hiring, and criminal justice, humans should remain in control. AI can provide recommendations — but a trained human must make the final call.
This approach helps prevent over-reliance on AI and creates accountability if something goes wrong.
Diverse development teams
When the people designing the system come from different backgrounds, they’re more likely to notice bias early. Inclusive teams lead to inclusive technology.
Some companies are also engaging external ethics panels and community feedback before deploying models at scale.
Regulation and responsibility: the role of government and industry
AI bias won’t be solved by developers alone. Governments and international bodies are stepping in to regulate and enforce ethical use.
- The EU’s AI Act classifies high-risk systems (e.g., medical or law enforcement AI) and requires transparency, human oversight, and non-discrimination.
- The U.S. White House Blueprint for an AI Bill of Rights outlines guidelines for safety, transparency, and fairness.
- Private companies are also establishing internal AI ethics teams and publishing annual bias audits.
We’re moving toward a world where ethical compliance will be a legal requirement, not just a branding strategy.
Final thoughts: building AI we can trust
Bias in AI is not just a technical problem — it’s a societal one. But with awareness, collaboration, and careful design, we can move toward systems that are more accurate, more inclusive, and more just.
The path forward requires both humility and innovation: the humility to admit our systems are flawed, and the innovation to make them better.
Every stakeholder — from data scientist to policymaker to user — plays a role in shaping AI’s impact. And if we take that responsibility seriously, the result will be technology that serves people, not statistics.
Want more insights like this?
Every week, we share practical breakdowns of the latest AI breakthroughs, ethical dilemmas, and real-world tools. Whether you’re a developer, business leader, or just curious about how AI is shaping society, we’ve got you covered.
Sign Up For Our Weekly Newsletter and Get Your FREE Ebook " AI For Everyone - Learn the Basics and Embrace the Future"
Clarity. Insight. No hype — just what you need to stay ahead of the curve.
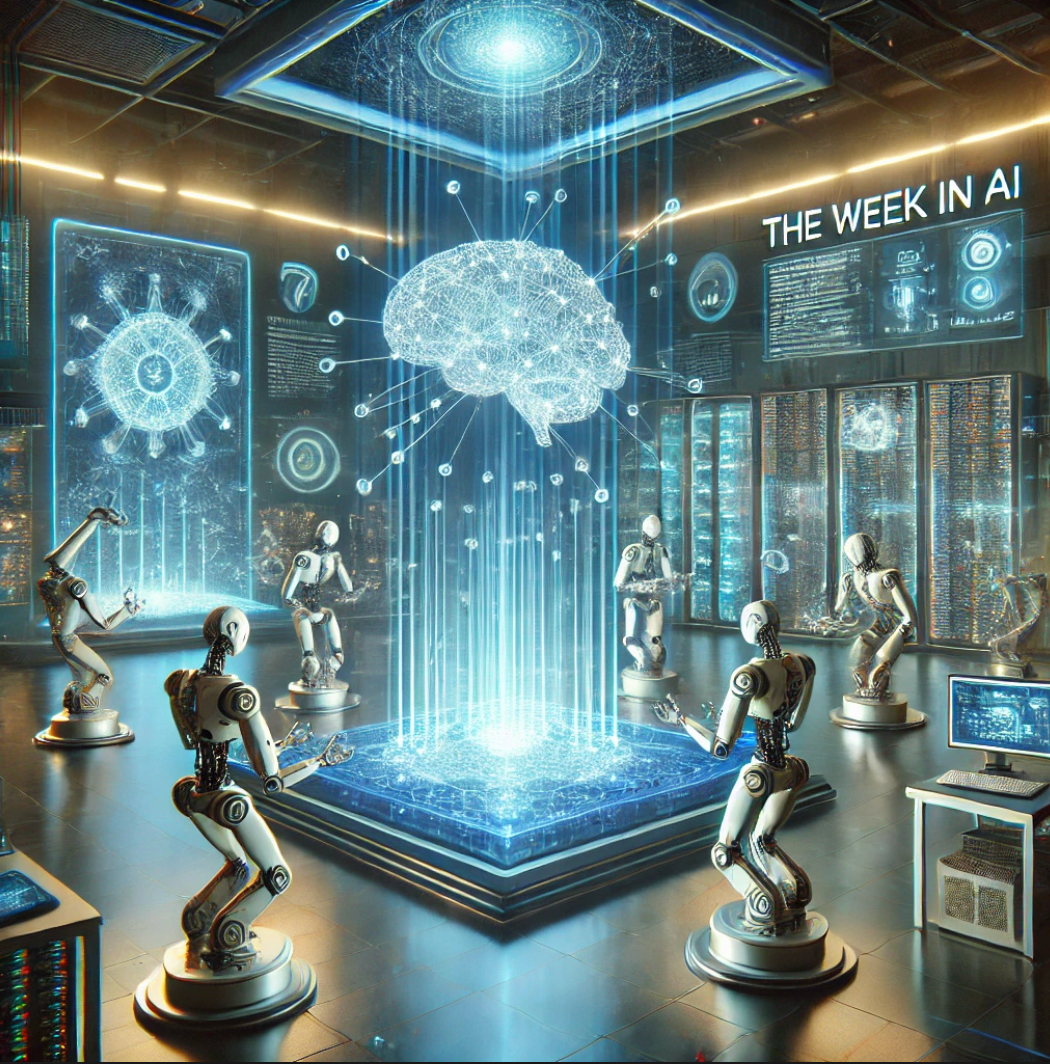