Protecting Privacy and Data Security in the Age of AI
Navigating the Digital Frontier
In an era where artificial intelligence is reshaping our world at an unprecedented pace, the need to safeguard our personal information has become more critical than ever before. As we entrust more aspects of our lives to smart devices and AI-powered services, a crucial question emerges: How can we ensure our privacy remains intact while benefiting from these technological advancements? Let's embark on a comprehensive exploration of the complex landscape of data protection in the AI age and discover how cutting-edge solutions are rising to meet this challenge.
The AI Privacy Paradox: A Deep Dive
Artificial Intelligence technologies offer a world of possibilities, revolutionizing industries and enhancing our daily lives. However, they also create new vulnerabilities that we must address. Let's take a closer look at the pros and cons of AI in relation to privacy and data security:
Pros:
Enhanced security measures:
- AI can detect and respond to threats faster than humans, often in real-time.
- Machine learning algorithms can identify patterns indicative of cyberattacks or data breaches that might be imperceptible to human analysts.
- AI-powered systems can continuously adapt to new threats, improving overall security posture.
Personalized experiences:
- AI tailors services to individual needs, improving user satisfaction and efficiency.
- Recommendation systems powered by AI can provide highly relevant content and product suggestions.
- Personalized healthcare applications can offer more accurate diagnoses and treatment plans.
Efficient data processing:
- AI can handle vast amounts of data, enabling better decision-making across various sectors.
- Big data analytics powered by AI can uncover insights that drive innovation and improve services.
- Automated data processing reduces human error and increases productivity.
Improved fraud detection:
- AI algorithms can identify fraudulent activities in financial transactions with high accuracy.
- Anomaly detection systems can flag unusual behavior patterns that may indicate security breaches.
Advanced biometric authentication:
- AI-driven biometric systems (facial recognition, fingerprint analysis) can provide more secure and convenient authentication methods.
Cons:
Data collection concerns:
- AI systems often require extensive personal data to function effectively, raising questions about data ownership and consent.
- The centralization of large datasets creates attractive targets for cybercriminals.
- Users may not fully understand the extent of data collection or how their information is being used.
Potential for misuse:
- Powerful AI tools could be exploited by bad actors if not properly secured.
- Deepfake technology, powered by AI, can be used to create convincing but false audio and video content.
- AI-driven surveillance systems could be used to infringe on personal privacy and civil liberties.
Lack of transparency:
- Many AI algorithms operate as "black boxes," making it difficult to understand how decisions are made.
- This opacity can lead to unintended biases and discrimination in AI-driven decision-making processes.
- Lack of explainability in AI systems can complicate compliance with data protection regulations.
Data breaches and AI-powered attacks:
- As AI systems become more prevalent, they also become targets for sophisticated cyberattacks.
- AI can be used to automate and scale up hacking attempts, potentially leading to more frequent and severe data breaches.
Privacy erosion through data inference:
- AI's ability to infer sensitive information from seemingly innocuous data can lead to unintended privacy violations.
- Cross-referencing multiple data sources can reveal more about individuals than they intended to share.
Key Features of Modern AI Privacy Solutions: A Comprehensive Overview
To address these challenges, innovative companies are developing robust privacy protection tools. Here's an in-depth look at some key features to consider:
End-to-end encryption:
- Ensures data remains unreadable to unauthorized parties during transmission and storage.
- Implements strong cryptographic algorithms to protect data at rest and in transit.
- Utilizes perfect forward secrecy to minimize the impact of potential key compromises.
Federated learning:
- Allows AI models to learn from decentralized data sources without compromising individual privacy.
- Enables collaborative model training across multiple parties without sharing raw data.
- Reduces the need for centralized data storage, minimizing potential attack surfaces.
Differential privacy:
- Adds carefully calibrated "noise" to datasets to protect individual records while maintaining overall accuracy.
- Provides mathematical guarantees about the level of privacy protection offered.
- Allows for privacy-preserving data analysis and machine learning on sensitive datasets.
Homomorphic encryption:
- Enables computations on encrypted data without decrypting it first.
- Allows for secure outsourcing of computations to untrusted third parties.
- Supports privacy-preserving machine learning and data analytics in cloud environments.
Data minimization:
- Collects only essential information, reducing the risk of exposure and complying with data protection regulations.
- Implements privacy-by-design principles in AI system development.
- Regularly audits and purges unnecessary data to minimize potential privacy risks.
Secure multi-party computation:
- Allows multiple parties to jointly compute functions over their inputs while keeping those inputs private.
- Enables privacy-preserving collaborations and data sharing between organizations.
Zero-knowledge proofs:
- Provides a way to prove knowledge of a secret without revealing the secret itself.
- Useful for privacy-preserving authentication and verification in AI systems.
Blockchain-based data governance:
- Utilizes distributed ledger technology to create tamper-evident audit trails for data access and usage.
- Implements smart contracts to automate and enforce data protection policies.
Privacy-preserving record linkage:
- Enables the combination of datasets from different sources without exposing individual records.
- Crucial for privacy-respecting data integration in healthcare and other sensitive domains.
Synthetic data generation:
- Creates artificial datasets that maintain statistical properties of real data without exposing individual records.
- Useful for training AI models when access to real data is limited due to privacy concerns.
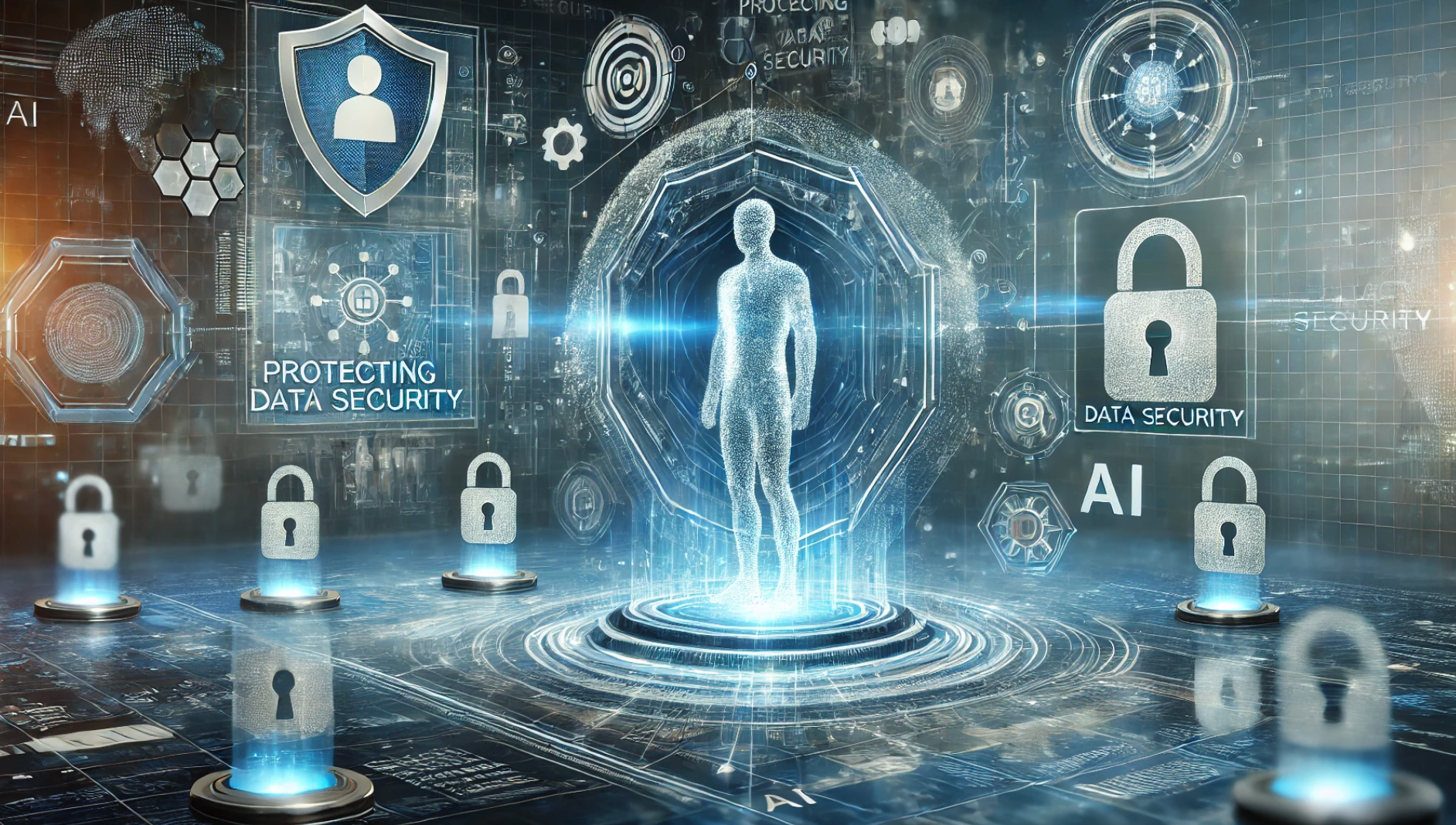
Real-World Examples: AI Privacy in Action
Let's examine how these features are being implemented in practice across various industries:
Apple's privacy-preserving face recognition:
- Uses on-device processing to avoid storing facial data in the cloud.
- Implements differential privacy techniques to improve recognition algorithms without compromising user privacy.
Google's RAPPOR (Randomized Aggregatable Privacy-Preserving Ordinal Response):
- Applies randomization techniques to protect user privacy in large-scale data collection.
- Allows for population-level insights without exposing individual user data.
Microsoft's Confidential Computing:
- Utilizes hardware-based trusted execution environments to process sensitive data securely.
- Enables secure cloud computing on encrypted data, even in untrusted environments.
IBM's Fully Homomorphic Encryption toolkit:
- Provides developers with tools to implement homomorphic encryption in their applications.
- Enables secure data processing and analytics on encrypted data.
Nvidia's Federated Learning Framework:
- Allows AI models to be trained across multiple edge devices without centralizing sensitive data.
- Particularly useful in healthcare for training models on distributed patient data.
Facebook's Privacybot:
- An AI-powered tool that helps users understand and manage their privacy settings.
- Uses natural language processing to interpret user preferences and automate privacy controls.
Amazon's AWS Macie:
- Uses machine learning to automatically discover, classify, and protect sensitive data in the cloud.
- Provides continuous monitoring and risk assessments for data privacy and security.
Inpher's Secret Computing platform:
- Enables privacy-preserving machine learning and analytics across multiple data sources.
- Utilizes advanced cryptographic techniques to perform computations on encrypted data.
OpenMined's PySyft:
- An open-source library for secure and private deep learning.
- Implements federated learning, differential privacy, and encrypted computation techniques.
Oasis Labs' Confidential Cloud Computing Platform:
- Combines blockchain technology with trusted execution environments for secure and private cloud computing.
- Enables privacy-preserving smart contracts and decentralized applications.
The PrivacyShield AI Suite: Your Digital Fortress
Imagine a world where you can harness the power of AI without compromising your personal information. That's the promise of PrivacyShield AI Suite, a comprehensive solution designed to protect your digital life in the age of artificial intelligence.
With PrivacyShield, you'll enjoy:
- Military-grade encryption for all your data, both at rest and in transit
- AI-powered threat detection that evolves with emerging risks, staying one step ahead of potential attackers
- Granular control over what information you share and with whom, putting you in charge of your digital footprint
- Seamless integration with your favorite apps and services, ensuring privacy doesn't come at the cost of convenience
- Federated learning capabilities that allow you to benefit from AI improvements without exposing your raw data
- Differential privacy implementations that add an extra layer of protection to your sensitive information
- Homomorphic encryption features for secure data processing in untrusted environments
- Blockchain-based audit trails that provide transparency and accountability for all data access and usage
- Synthetic data generation tools for privacy-preserving analytics and machine learning
- Regular privacy impact assessments and automated compliance checks for peace of mind
PrivacyShield AI Suite is more than just a privacy tool – it's a comprehensive ecosystem designed to empower you in the digital age. Our team of world-class cryptographers, AI experts, and privacy advocates work tirelessly to stay ahead of emerging threats and regulatory requirements.
But don't just take our word for it. Try PrivacyShield AI Suite free for 30 days and experience the peace of mind that comes with knowing your data is truly secure. Our intuitive interface and step-by-step tutorials make it easy to take control of your digital privacy, even if you're not a tech expert.
Click here to start your trial and join the thousands of individuals and businesses who have already taken control of their digital privacy with PrivacyShield. Don't wait until it's too late – protect your data today and enjoy the benefits of AI with confidence!
Stay Informed, Stay Protected: Join Our Privacy-First Community
The world of AI and privacy is constantly evolving, with new challenges and solutions emerging every day. To keep up with the latest developments and receive exclusive tips on protecting your digital life, sign up for our newsletter.
Remember, in the age of AI, privacy isn't just a right – it's a responsibility. Take control of your digital destiny with PrivacyShield AI Suite and our privacy-first community. Your future self will thank you.
Sign Up For Our Weekly Newsletter and Get Your FREE Ebook " AI For Everyone - Learn the Basics and Embrace the Future"